Manuel Gomez-Rodriguez awarded ERC Consolidator Grant
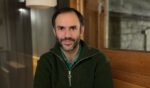
Manuel Gomez-Rodriguez, head of the MPI-SWS Human-Centric Machine Learning group, has been awarded an ERC Consolidator Grant for his project "Counterfact: Counterfactuals in Minds and Machines." This project will receive circa 2 million euros over a period of 5 years, starting directly after Manuel's ERC Starting Grant project on "Human-Centered Machine Learning" wraps up next year.
In the most recent round for Consolidator Grants, over 2300 research proposals were submitted to the ERC.
...Manuel Gomez-Rodriguez, head of the MPI-SWS Human-Centric Machine Learning group, has been awarded an ERC Consolidator Grant for his project "Counterfact: Counterfactuals in Minds and Machines." This project will receive circa 2 million euros over a period of 5 years, starting directly after Manuel's ERC Starting Grant project on "Human-Centered Machine Learning" wraps up next year.
In the most recent round for Consolidator Grants, over 2300 research proposals were submitted to the ERC. The sole selection criterion is scientific excellence. This year, less than 15% of all ERC Consolidator Grant applicants across all scientific disciplines received the award, with only 16 awardees in Computer Science across all of Europe!
Summary of the Counterfact project proposal
Reasoning about what might have been, about alternatives to our own pasts, is a landmark of human intelligence. This type of reasoning, called counterfactual reasoning, is often evaluative, specifying alternatives that are in some sense better or worse than our past reality, and has been shown to play a significant role in the ability that humans have to learn from limited past experience and improve their decision making skills over time. In recent years, there has increasing excitement about the potential of machine learning models and algorithms to support human decision making in a variety of high-stakes domains such as medicine, education or science. However, these models and algorithms have been traditionally unable to perform, nor benefit from, counterfactual reasoning. In this project, our goal is to bridge this gap.
We will develop machine learning models and algorithms for automated decision support that are able to perform and benefit from counterfactual reasoning in multiple ways. For example, they will perform counterfactual reasoning about human behavior in order to anticipate how humans incorporate algorithmic advice into their decisions. This will enable a new generation of decision support systems that can only increase and never decrease the average quality of human decisions. Moreover, they will use the structural similarities and shared properties across different counterfactual decision making scenarios to significantly reduce their computational and data requirements. In addition, these models and algorithms will also help humans learn from their own past decisions by identifying alternative decisions that would have led to better outcomes. Finally, we will perform large-scale human subject studies with both laypersons and experts to evaluate their effectiveness in a wide variety of decision making tasks.