Francis' group releases SynDiffix, the world's most accurate synthetic data generator
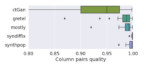
Paul Francis's Open Diffix project has released SynDiffix, an open-source Python package that generates statistically accurate, privacy preserving synthetic data from structured data. Using SynDiffix, a data owner can safely share data while retaining most of the statistical properties of the original data. Analysts can work with the synthetic data as though it were the original.
Using the novel techniques of sticky noise and range snapping, ...
Using the novel techniques of sticky noise and range snapping, ...
Paul Francis's Open Diffix project has released SynDiffix, an open-source Python package that generates statistically accurate, privacy preserving synthetic data from structured data. Using SynDiffix, a data owner can safely share data while retaining most of the statistical properties of the original data. Analysts can work with the synthetic data as though it were the original.
Using the novel techniques of sticky noise and range snapping, SynDiffix breaks new ground in data accuracy. It is 10 to 100 times more accurate than the open source tool CTGAN, and 5 to 10 times more accurate than the best commercial synthetic data generators. This makes SynDiffix particularly well suited to descriptive analytics like histograms, heatmaps, averages and standard deviations, column correlations, and so on. Like other tools, however, it can also be used for ML modeling. Francis is hopeful that SynDiffix will find wide practical use as well as motivate more research on synthetic data.
Using the novel techniques of sticky noise and range snapping, SynDiffix breaks new ground in data accuracy. It is 10 to 100 times more accurate than the open source tool CTGAN, and 5 to 10 times more accurate than the best commercial synthetic data generators. This makes SynDiffix particularly well suited to descriptive analytics like histograms, heatmaps, averages and standard deviations, column correlations, and so on. Like other tools, however, it can also be used for ML modeling. Francis is hopeful that SynDiffix will find wide practical use as well as motivate more research on synthetic data.