Viktor Vafeiadis awarded ERC Consolidator Grant
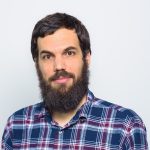
Viktor Vafeiadis, head of the MPI-SWS Software Analysis and Verification group, has been awarded an ERC Consolidator Grant. Over the next five years, his project "PERSIST: A Semantic Foundation for Persistent Programming" will receive almost 2 million euros, which will allow the group to develop rigorous formal foundations for programs interacting with non-volatile memory. Read more about the PERSIST project below.
One of the other recipients of an ERC Consolidator Grant this year is an MPI alumnus: Neel Krishnaswami was an MPI-SWS postdoc with Derek Dreyer, ...
One of the other recipients of an ERC Consolidator Grant this year is an MPI alumnus: Neel Krishnaswami was an MPI-SWS postdoc with Derek Dreyer, ...
Viktor Vafeiadis, head of the MPI-SWS Software Analysis and Verification group, has been awarded an ERC Consolidator Grant. Over the next five years, his project "PERSIST: A Semantic Foundation for Persistent Programming" will receive almost 2 million euros, which will allow the group to develop rigorous formal foundations for programs interacting with non-volatile memory. Read more about the PERSIST project below.
One of the other recipients of an ERC Consolidator Grant this year is an MPI alumnus: Neel Krishnaswami was an MPI-SWS postdoc with Derek Dreyer, and he is currently a faculty member at Cambridge.
ERC grants are the most prestigious and the most competitive European-level awards for ground-breaking scientific investigations. This year, less than 14% of all ERC Consolidator Grant applicants across all scientific disciplines received the award, with only 15 awardees in Computer Science across all of Europe! The ERC Consolidator Grant offers funding for researchers with 7 to 12 years of experience after achieving a PhD. You can find more information about ERC Consolidator Grants awarded this year at https://erc.europa.eu/news/CoG-recipients-2020.
The European Research Council (ERC) is a pan-European funding body that supports cutting-edge research. It offers funding for groundbreaking research projects of the highest scientific quality across Europe, across all research areas. Talented researchers from all over the world can receive funding for excellent research in Europe.
The PERSIST Project
Non-volatile memory (NVM) is an emerging technology that provides orders of magnitude faster access to persistent storage (which preserves its contents after a crash or a power failure) than hard disks. As such, it is expected to radically change how modern applications manage storage, moving away from traditional block-structured file systems to in-memory persistent data structures.
The problem with NVM, however, is that its programming model is standing on very shaky foundations. The persistency semantics of the mainstream architectures is unclear and full of counterintuitive behaviours, which makes writing correct NVM programs a very challenging task.
The project's goal is to develop a solid mathematical basis for determining the semantics of NVM programs and for reasoning about their correctness. More specifically, the plan is to produce:
One of the other recipients of an ERC Consolidator Grant this year is an MPI alumnus: Neel Krishnaswami was an MPI-SWS postdoc with Derek Dreyer, and he is currently a faculty member at Cambridge.
ERC grants are the most prestigious and the most competitive European-level awards for ground-breaking scientific investigations. This year, less than 14% of all ERC Consolidator Grant applicants across all scientific disciplines received the award, with only 15 awardees in Computer Science across all of Europe! The ERC Consolidator Grant offers funding for researchers with 7 to 12 years of experience after achieving a PhD. You can find more information about ERC Consolidator Grants awarded this year at https://erc.europa.eu/news/CoG-recipients-2020.
The European Research Council (ERC) is a pan-European funding body that supports cutting-edge research. It offers funding for groundbreaking research projects of the highest scientific quality across Europe, across all research areas. Talented researchers from all over the world can receive funding for excellent research in Europe.
The PERSIST Project
Non-volatile memory (NVM) is an emerging technology that provides orders of magnitude faster access to persistent storage (which preserves its contents after a crash or a power failure) than hard disks. As such, it is expected to radically change how modern applications manage storage, moving away from traditional block-structured file systems to in-memory persistent data structures.
The problem with NVM, however, is that its programming model is standing on very shaky foundations. The persistency semantics of the mainstream architectures is unclear and full of counterintuitive behaviours, which makes writing correct NVM programs a very challenging task.
The project's goal is to develop a solid mathematical basis for determining the semantics of NVM programs and for reasoning about their correctness. More specifically, the plan is to produce:
- Formal persistency models for mainstream hardware architectures,
- Formal persistency models for mainstream programming languages,
- Firmly-grounded higher-level abstractions to ease persistent programming, and
- Effective testing and verification techniques for persistent programs (e.g., program logics and model checking).